Simplifying skills intelligence platforms - introducing the breadth vs depth concept for skills
Buyers can consider many types of skills intelligence platforms, and the underlying technology can differ dramatically. So, how do we know which is best for our HR requirements?
2.png)
Our previous blog post explored the definition of skills intelligence and how platform providers help organisations solve their skills challenges.
However, one significant obstacle to this is the fragmented skills intelligence marketplace.
The marketplace is crowded, and while some point solution providers claim they are comprehensive, no platform covers all skills, roles, and applications.
As Josh Bersin has noted: “We have yet to find a company that uses 1 platform for everything. So while we may, at some point, find a single ‘Skills Cloud’ capable of storing every skill for every job in the company, that goal has yet to be achieved.”
With that in mind, let’s summarise how skills intelligence platforms differ.
How they organise skills demand data
Skills intelligence platforms can use 1 of 2 ways to organise demand-side skills data and create taxonomies.
- Inference. Platforms using inference to understand skills demand scrape massive amounts of internal and external skills data from sources such as CVs and job descriptions. They then use machine learning to determine the ontological relationships between skills and skills groups.
- Design. This approach uses input from company stakeholders (such as hiring managers and recruiting teams) to create a taxonomy that defines skills and maps them to roles and organisations.
How they source skills supply data
Skills intelligence platforms can pull from 3 sources to create skills profiles for individuals.
- Self-reporting. Self-reporting can be as basic as looking at the skills an individual has tagged themselves with on their LinkedIn profile or creating a spreadsheet and having a manager verify it.
- Inference. Similar to how inference is used to create role skill taxonomies, this approach scrapes secondary data from various sources (e.g. CVs and performance reviews) and infers employee skills.
- Verification. Due to their low cost, self-reporting and inference can cover a wide range of skills. However, they lack accuracy. If you want a verified skills profile that includes proficiency, you’ll need to use a mix of data sources, including manager/peer feedback, interviews, credentials, and assessments.
How to understand skills intelligence platforms
So, there are many options for buyers to consider, and it can quickly become confusing.
However, we can simplify our understanding of the skills intelligence landscape by categorising the underlying technology into 2 camps: breadth platforms and depth platforms.
Breadth platforms
Breadth platforms identify skills. On the skills demand side, they aggregate labour market data to label and define skills, create skills taxonomies, and identify skills adjacencies. On the skills supply side, they can build individuals’ skills profiles by scraping data from job descriptions, CVs, and technology platforms such as Human Capital Management systems.
Key things to note:
- They use AI to make inferences about skills.
- They are more focused on speed than on accuracy.
- They do not cover skills proficiency.
Some examples of breadth players include Lightcast, a provider of integrated jobs, skills, roles, and occupational data, and Eightfold.ai, a talent marketplace and talent management platform.
Depth platforms
Depth platforms verify skills. They allow people to generate granular and accurate data about skills in an efficient way, augmented by technology. For instance, depth platforms may use a combination of online assessments, manager/peer feedback, self-assessment, and observation to accurately and efficiently verify technical and behavioural skills.
On the skills demand side, these platforms create role skills profiles detailing the technical and behavioural skills required for success and at what proficiency level.
Key things to note:
- They use a combination of humans and technology to verify skills.
- They ensure greater accuracy.
- They cover technical and behavioural skills, as well as their proficiency.
An example of a depth player is Spotted Zebra, which provides high-confidence, granular skills data to support hiring, reskilling, and development decision-making.
What can we do with this knowledge?
Differentiating between depth platforms and breadth platforms helps us simplify the complex skills intelligence marketplace. Crucially, because depth and breadth platforms each have specific strengths, this distinction also helps us identify which platform is best suited to address our HR priorities.
To learn more about this, download our guide. It will help you understand which HR decisions are best served by depth platforms and which by breadth platforms.
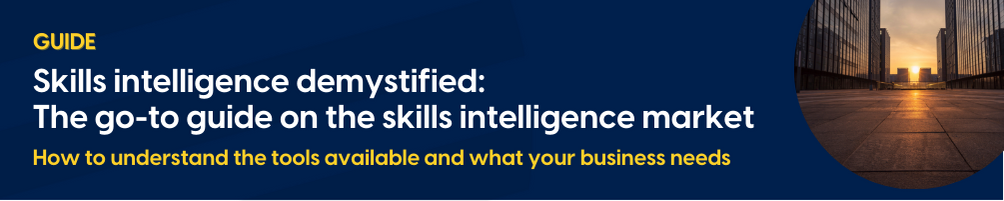
FAQs
1. How do skills intelligence platforms address the challenge of the fragmented marketplace, and what are the key differences between breadth and depth platforms in terms of their approach to organising skills data?
Skills intelligence platforms address the challenge of the fragmented marketplace by offering different approaches to organising skills data. Breadth platforms typically use inference, scraping massive amounts of data from various sources like CVs and job descriptions, and then employing machine learning to understand ontological relationships between skills. Depth platforms, on the other hand, focus on verification, using a combination of methods such as online assessments, manager/peer feedback, and observation to ensure the accuracy and granularity of skills data.
2. What are the advantages and disadvantages of using inference versus design in organising demand-side skills data, and how does each approach impact the accuracy and comprehensiveness of skills intelligence platforms?
The advantages of using inference in organising demand-side skills data include the ability to quickly aggregate labour market data and create skills taxonomies. However, this approach may lack accuracy compared to design, where input from company stakeholders is used to define skills and map them to roles and organisations. While inference can cover a wide range of skills, it may lack precision. Design, though potentially more accurate, requires more human input.
3. In what ways do breadth and depth platforms differ in their sourcing of skills supply data, and how does this difference influence the accuracy and reliability of skills profiles generated by these platforms?
Breadth platforms typically source skills supply data through self-reporting and inference. Self-reporting involves individuals tagging their skills on platforms like LinkedIn. Inference scrapes secondary data from sources like CVs and performance reviews. While these methods are cost-effective and can cover a wide range of skills, they may lack accuracy. Depth platforms, however, prioritise accuracy by combining human input and technology to verify skills through methods like online assessments, manager/peer feedback, and observation. This ensures greater precision in skill profiles, particularly regarding proficiency levels.